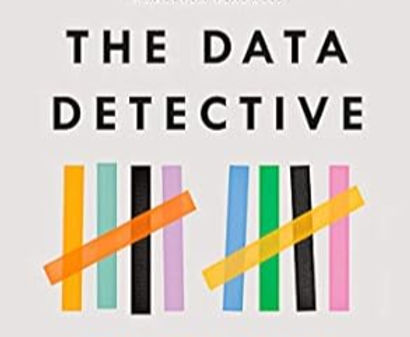
Summary
Most of the data-based conclusions we read in popular media that suggest a cause-and-effect relationship are either inaccurate or very premature. There are a large number of biases that can cause this to happen, including:
The Saliency Bias - Only reporting the results of surprising (click-bait) studies, and ignoring unsurprising or unremarkable conclusions
Cherry-Picking Data - Only using the data that confirm pre-existing beliefs or desires
Sampling Bias - Pretending that study volunteers or survey respondents are representative of the general population
The author has 2 goals:
To educate readers about these common biases
To leave readers feeling optimistic about this new knowledge (vs. cynical about the ocean of bad reporting common in popular media)
Does the author succeed in educating the reader?
The biases he focuses on are indeed pervasive (i.e. great selections). His explanations are easy-to-understand, and his examples well-illustrate the potential consequences of falling victim to those biases. I not only feel like I’ve learned a lot, but that I’ll actually remember most of his examples.
Notably, you don’t have to read the entire 280-page book to greatly benefit from it.
Each chapter explores a different bias, and how ignoring that bias has led to ridiculous– and sometimes catastrophic– consequences. The final chapter recaps the 10 biases. This means that you can skip to the last chapter, and then visit individual earlier ones as desired.
In the future, I’m probably going to recommend individual chapters to different coaching clients, and I’m grateful that the author made that feasible.
Does the author succeed in leaving the reader optimistic?
Not entirely, but I think if you read the book and my addendum below, then it might:
When I first learned about these biases, it evoked a sense of futility; proving a cause-and-effect relationship through data seems nearly impossible. Then I had an epiphany.
Popular media often make premature claims of cause-and-effect relationships because humans crave certainty. Certainty means safety. Though momentarily comforting, this media trend has created unrealistic expectations about the minimum level of certainty we need to consider data interesting.
In reality, if we make 20 choices based on data showing a weak correlation between Choice X and Positive Outcome Y, our lives will almost certainly get better, even if half those correlations end up being coincidence.
I now feel inoculated against clickbait claims and more comfortable taking action with imperfect knowledge. In short, I am now better able to navigate the world.
My General Recommendations (inspired by this book):
When you see an interesting conclusion, focus on the data and methodology used- but ignore the stated conclusion
Read the text, examine the evidence, and decide whether it’s persuasive enough to convince you of any meaningful conclusion. If you ignore the stated conclusion, then you won’t be angry if it turns out to be misleading or baseless.
Assume positive intent
Most humans generally tend to be well-intentioned. However, we’re wired with 100s of cognitive biases. We all make mistakes– especially when we want something to be true (e.g. because it benefits us).
Even when there isn't positive intent, there's usually little consequence to remaining optimistic– as long as you remain laser-focused on the evidence. This will help protect you from cynicism about humanity (it's been highly effective for me, at least).